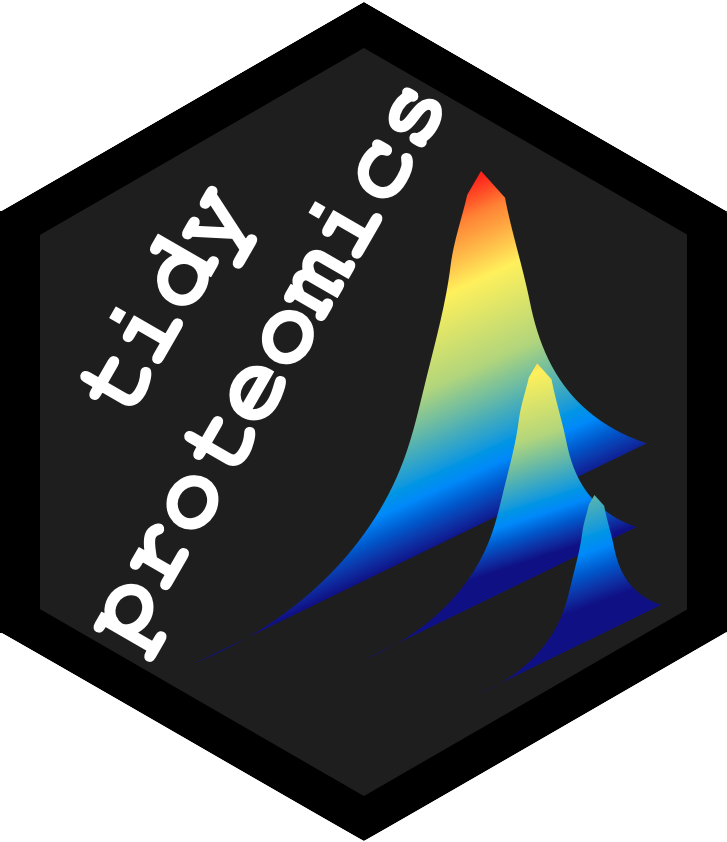
Enrichment
enrichment.Rmd
Term Enrichment
Available Methods
GSEA |
Korotkevich G, Sukhov V, Sergushichev A (2019). “Fast gene set enrichment analysis.” bioRxiv. doi:10.1101/060012, http://biorxiv.org/content/early/2016/06/20/060012. |
Wilcoxon Rank Sum |
H. B. Mann, D. R. Whitney “On a Test of Whether one of Two Random Variables is Stochastically Larger than the Other,” Ann. Math. Statist. 18(1), 50-60, (March, 1947) [R::stats::wilcoxon] |
Fisher’s Excact Test |
Fisher, R. A. “On the Interpretation of χ2 from Contingency Tables, and the Calculation of P.” Journal of the Royal Statistical Society, vol. 85, no. 1, 1922, pp. 87–94. [R::stats::fisher.test] |
Term enrichment analysis is possible proceeding an expression
analysis with the enrichment()
function, employing either
the GSEA algorithm, a simple Wilcoxon rank sum
comparison, or Fisher’s Exact Test and can be visualized with
the plot_enrichment()
function. Both GSEA and
Wilcoxon will consider genes of a given term significant if
those genes are skewed mostly up or down. The Fisher’s Exact
Test will consider genes of a given term significant if they are
“represented” more often than expected – this is the basis of
Over-Representation Analysis and will consider the
gene-set regardless of being skewed mostly up or down.
library("dplyr")
library("tidyproteomics")
rdata <- hela_proteins %>%
normalize(.method = 'linear') %>%
expression(knockdown/control) %>%
enrichment(knockdown/control,
.terms = 'biological_process',
.method = 'wilcoxon')
Exporting Results
The results of this analysis are stored in the tidyproteomics data-object and can easily be exported to save as a flat data table or used in an external down stream analysis.
rdata %>%
export_analysis(knockdown/control,
.analysis = 'enrichment',
.term = 'biological_process')
#> # A tibble: 13 × 5
#> annotation p_value adj_p_value enrichment size
#> <chr> <dbl> <dbl> <dbl> <int>
#> 1 conjugation 0.0000213 0.000277 0.0257 1227
#> 2 cell proliferation 0.000292 0.00350 -0.0704 301
#> 3 development 0.00653 0.0719 0.0326 879
#> 4 cellular component movement 0.0172 0.172 -0.0264 1015
#> 5 cell organization and biogenesis 0.0249 0.224 -0.00246 1373
#> 6 defense response 0.0590 0.472 0.00925 848
#> 7 cell differentiation 0.109 0.765 -0.0215 551
#> 8 metabolic process 0.151 0.907 -0.00572 3179
#> 9 coagulation 0.168 0.907 0.000746 961
#> 10 cell communication 0.441 1 -0.00345 157
#> # ℹ 3 more rows
The results can also have any other terms appended to the output, such as protein or gene_name, which may be useful for follow-on analyses.
rdata %>%
export_analysis(knockdown/control,
.analysis = 'enrichment',
.term = 'biological_process',
.append = 'gene_name')
#> # A tibble: 13 × 6
#> annotation p_value adj_p_value enrichment size gene_names
#> <chr> <dbl> <dbl> <dbl> <int> <chr>
#> 1 conjugation 2.13e-5 0.000277 0.0257 1227 DYNC1H1, …
#> 2 cell proliferation 2.92e-4 0.00350 -0.0704 301 PRKDC, HU…
#> 3 development 6.53e-3 0.0719 0.0326 879 PRKDC, FL…
#> 4 cellular component movement 1.72e-2 0.172 -0.0264 1015 PRKDC, PR…
#> 5 cell organization and biogen… 2.49e-2 0.224 -0.00246 1373 PRKDC, FL…
#> 6 defense response 5.90e-2 0.472 0.00925 848 PLEC, PRK…
#> 7 cell differentiation 1.09e-1 0.765 -0.0215 551 PLEC, DYN…
#> 8 metabolic process 1.51e-1 0.907 -0.00572 3179 PLEC, AHN…
#> 9 coagulation 1.68e-1 0.907 0.000746 961 PLEC, FAS…
#> 10 cell communication 4.41e-1 1 -0.00345 157 TLN1, DSP…
#> # ℹ 3 more rows